New video: DynaPopeX
In the DynaPopeX project, TNO and Zicht op Data focus on reducing poor air quality. By combining data, location, and human movement patterns in Eindhoven, they develop tangible measures to minimize people’s exposure to harmful particulate matter and limit potential health risks. This is crucial for the municipality of Eindhoven to understand the most significant impacts of air quality and improve the health of its residents.
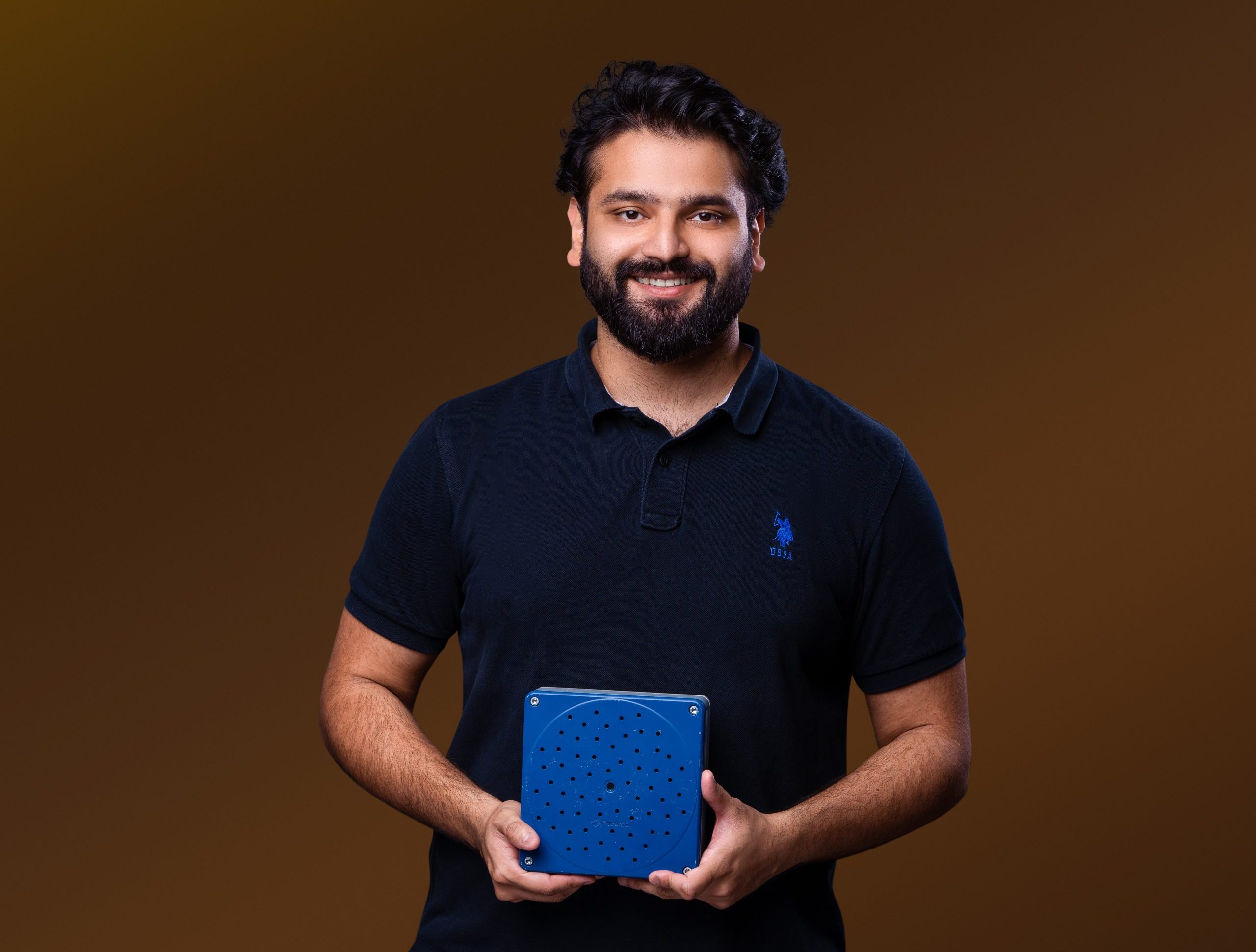
Tackling Europe’s Invisible Health Threat
Hi, my name is Devansh Kandpal. I’m 27 years old and I’m an Acoustics R&D Engineer at Sorama as well as an EngD trainee in Smart Buildings and Cities at TU/e.
Noise pollution is one of the largest contributors to poorer health in the European Union (EU). Disturbance of sleep due to environmental noise leads to the loss of millions of hours of sleep, which is directly responsible for added stress and poorer quality of life for residents of the EU. Addressing this issue, the OpenCall project VIPNOM has been conceived to develop advanced methods for noise measurement and visualization. VIPNOM, which stands for Virtual Position Noise Measurement, is a consortium between Sorama, TU Eindhoven and ReSound. It represents a convergence of diverse engineering disciplines, all focused on innovating scalable technologies that will revolutionize how noise is measured and visualized across various scenarios. I am pleased to undertake the assignment for my EngD traineeship as part of this project. The focus will be on resolving the inaccuracies identified in noise level recordings.
Revolutionizing stadiums and highways
VIPNOM’s breakthroughs have notably enhanced smart stadiums and highways. By integrating advanced, real-time audio capture algorithms, Sorama’s acoustic monitors can now effectively map noise data. This technology has transformed stadiums into intelligent arenas that utilize noise levels to foster positive fan conduct, thereby ensuring a secure and welcoming atmosphere.
In parallel, these principles have been adapted to monitor vehicular noise on highways. Sorama’s acoustic cameras serve as ‘noise radars’, pinpointing excessively loud vehicles to cultivate a more serene environment. These two applications have been adopted worldwide.
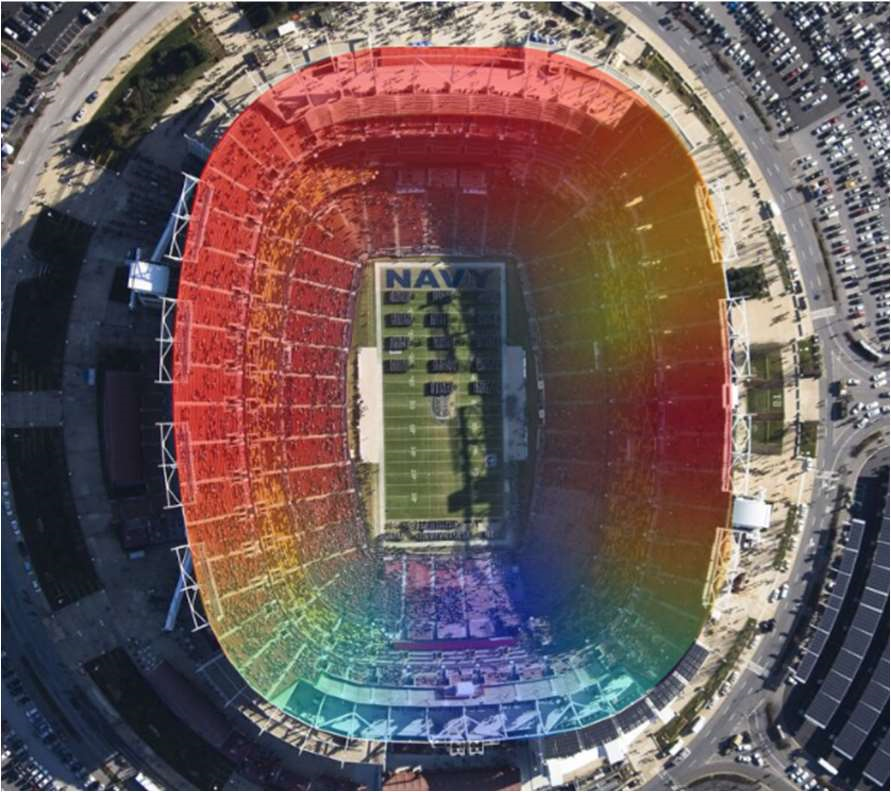
Enhancing accuracy through innovation
In some cases Sorama encounters inaccuracies in noise level recordings due to environmental variables and hardware constraints. The VIPNOM team is proactively addressing these issues through multiple approaches. These include designing predictive machine learning models to compensate for losses, using acoustic models to account for environmental conditions and how noise propagates in the same, and using physics-based compensation solutions for acoustic inaccuracy.
As part of this effort, I focus on crafting digital signal processing (DSP) algorithms essential for calibrating acoustic monitors in expansive venues like sports arenas. My work extends to creating simulations and visualizations that improve the spatial accuracy of beamformers within Sorama’s acoustic monitors, as well as developing transfer functions derived from acoustic modeling to further refine their performance.
A quieter world through acoustic innovation
VIPNOM aims to raise awareness about environmental noise and the health risks that it poses. We aspire to do this by creating new technologies that visualize noise in different ways. We aim to harness collected noise data to create solutions that make the world a quieter and healthier place to live in.
We aim to harness collected noise data to create solutions that make the world a quieter and healthier place to live in.
The Smart Heat Shed, but different
Hi, my name is Jamy de Lange. I am 21 years old, and I am currently finalizing my third year of my bachelor’s degree in Business Innovation.
At the end 2023 I worked on an OpenCall project with TNO and Emergo called the Smart Heat Shed (SHS). The SHS is an innovative storage facility that houses an installation for heating and cooling buildings. My challenge was make the SHS suitable for the housing corporations, so that it could be installed for many houses.
Getting started
The first step was to get a better understanding of de SHS by doing research. On the basis of these insights I was able to make questions for the housing corporations that were were already testing the SHS in practice (at a small scale), which is why I wanted to know about their experiences. The insight I gained from the interviews showed me that there was still some work to do to get de SHS suitable for the housing corporations.
After obtaining insights from my research I organized brainstorm sessions that were focused on the feedback from the housing corporations. I even wanted to try, if possible to use the SHS outside of the housing market (which is totally different than the purpose of which it is built). The results of this made me start to think about an interesting solution. Something that would bring music to everybody’s ears…
New insight
The concept I came up with is focused on applying the SHS to festivals. Further research revealed that some festivals use generators, consuming significant amounts of diesel. Lowlands, for example uses 120,000 liters of diesel to generate electricity. This is why I was certain I had to delve deeper in this direction.
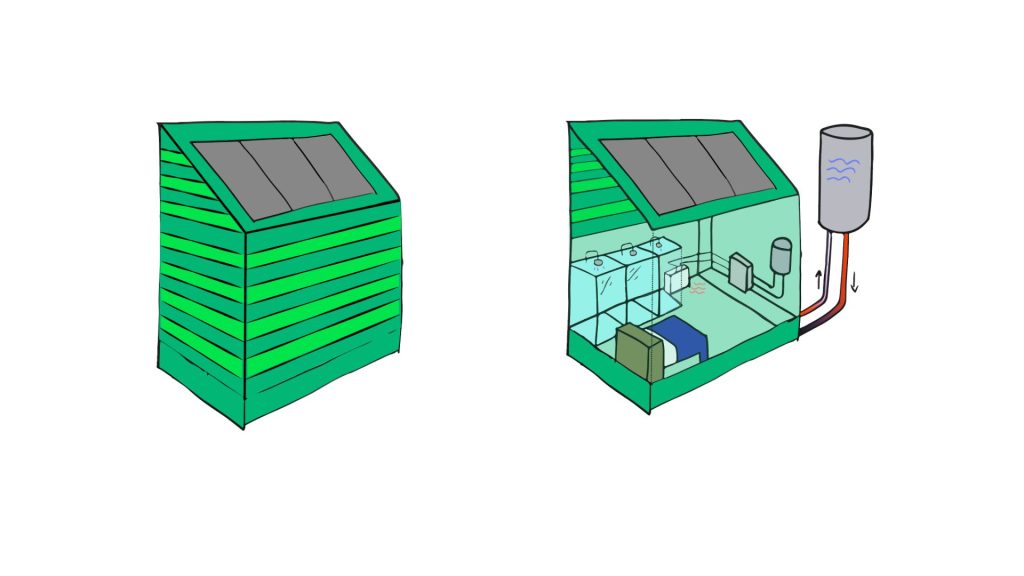
“Some festivals use generators, consuming significant amounts of diesel. Lowlands for example uses 120,000 liters of diesel to generate electricity.”
Smart Heat Shelter prototype
First I came in contact with a stakeholder at Emergo, just to make sure that my concept would be interesting for them. An enthusiastic reaction prompted me to focus on this concept. Later, I send an email to Lowlands, which, to my surprise, was submitted to the director. He provided feedback that gave me insight on adapting the concept for general application to festivals.
The Smart Heat Shelter
After the positive reactions I adjusted the concept based on the feedback given. After this step I tested it again until I had formed my final concept (see prototype picture). I am proud to present to you: The Smart Heat Shelter. This concept allows festivals to lease a certain number of sheds, which can be placed at an available spot. Once installed, the sheds use sunlight to produce heat, which can be used for showers or to heat the sheds when used as accommodation. . The sheds are in different varieties for guests to enjoy different types of stay.
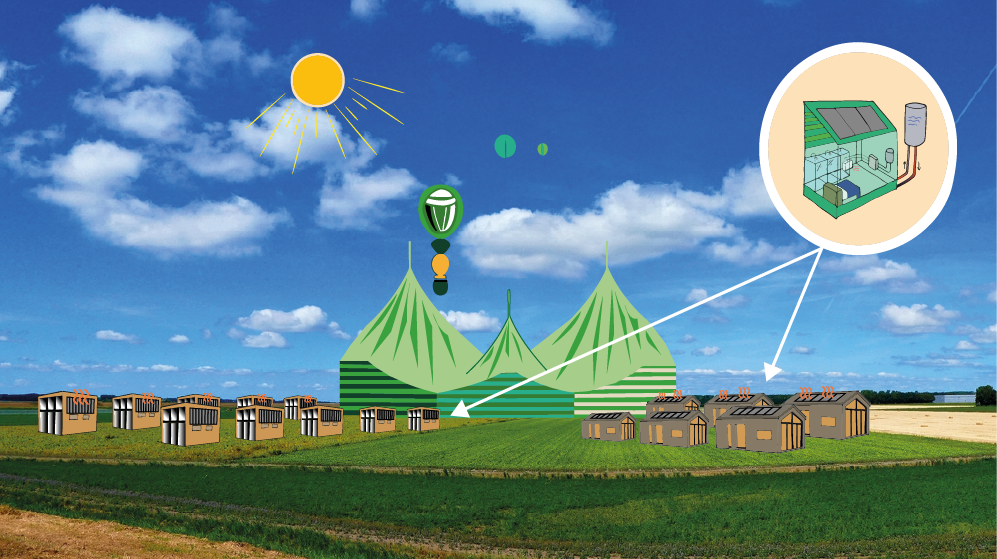
Recently, I engaged with numerous specialists across different sectors, seeking guidance from a business mentor and consulting professionals in eco-friendly (residential) building. Through these discussions, I’m refining my concept to make sure it’s ready for the marketplace.
Should you have any inquiries or suggestions after perusing this article, don’t hesitate to email me at: j.d.lange2@tue.nl
New project videos: POWERLIFT
The OpenCall project Powerlift focuses on enhancing battery packs for unmanned aerial vehicles used in transportation, inspection, emergency services, and security. Through this project, the partners LeydenJar, Tulip Tech, and Wingtra aim to increase the deployment of drones in emergency situations, including organ transplantation, blood transport, professional assistance over long distances, and security purposes.
Battery technology for the future
At LeydenJar, we are working on battery technology for the future. At the moment our battery for a drone can store 70% more energy. Drones can now flying longer distances, lifting off with ease, and exploring more locations. Through our collaboration at Eindhoven Engine with Tulip Tech and Wingtra, we are creating a modular design that allows customizable battery capacity and power—whether it’s a compact pack or a robust one, tailored precisely to each drone’s requirements.
Battery systems with the highest energy density
In project PowerLift we, Leydenjar, Tulip Tech and Wingtra, develop battery systems for industrial drones. As a partner within this project Tulip Tech builds battery systems with the highest specific energy density in the world. That means that the batteries have two to three times more energy per kilogram.
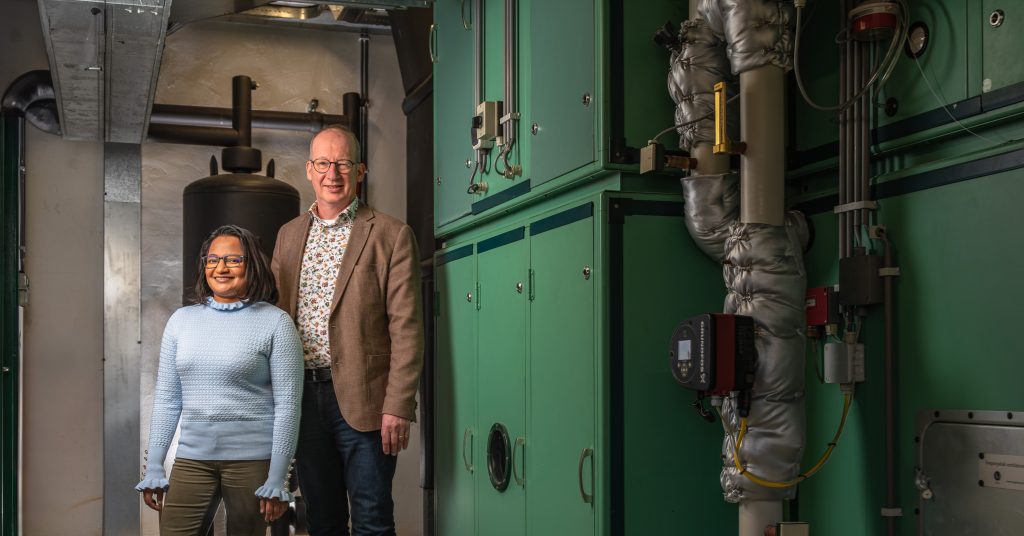
“I never saw myself working at Kropman,” Shalika Walker confesses. “And honestly, I might not have hired Shalika,” Joep van der Velden admits, seated beside her. “But how the OpenCall of Eindhoven Engine changed things. We’ve now created a new position within Kropman specifically to bring Shalika on board,” says Joep with a big smile.
We are at one of the offices of Kropman. This company designs, builds, maintains, and manages building installations, including climate control. “Kropman, rooted in construction, is traditionally less dynamic compared to sectors like semiconductors or the medical industry. So, working here was not on my radar,” Shalika shares.
Kropman’s Workspace: More Than Meets the Eye
“Welcome to our living lab,” Joep announces as he leads me into a spacious, open-plan office. At first glance, it looks ordinary, with dark gray carpets, white adjustable desks, and black office chairs. Joep notices my lukewarm reaction and gives a knowing sigh, directing my attention upward. “The real innovation is actually hidden above us in the ceiling.” I follow his gaze to a beige suspended ceiling, and he points out a translucent cap. “Those are sensors. They allow us to monitor and adapt the office environment, responding to the current scenario or the presence of workers.”
At Kropman, the pride lies in being system integrators. We source pumps from one company, sensors from another, and piping and operating systems from yet another. Our goal is to seamlessly blend these components, providing our customers with a unified operating system that manages lighting, climate control, and solar panels, among other things. This integration is meticulously crafted to enhance the experience for our clients’ employees, focusing on maximal efficiency and environmental sustainability, using as little energy as possible.
Embracing Open Collaboration for Innovation
Creating an integrated system might seem straightforward, but it’s a challenging endeavor. We’re in a field dominated by heavyweights like Siemens, Honeywell, and Signify, each offering products with proprietary systems and unique data outputs, typically closed to external systems. To navigate this, we developed our software to manage and optimize the diverse systems a client might have. However, the need for a testing ground became apparent. Clients often hesitate to open their buildings for experimental setups; hence, we transformed our Breda office into a living lab.
True partnership proved elusive until we found a more receptive community in the Brainport region.
We strongly believe in an open environment for research and development. Our efforts to collaborate with universities and companies across and beyond Europe, however have been met with limited success. True partnership proved elusive until we found a more receptive community in the Brainport region. This shares a work and research philosophy similar to our own company’s, plus communication is direct and efficient, enabling us to quickly and easily connect with each other. This welcoming atmosphere was pivotal when Wim Zeiler, a former colleague now with Eindhoven University of Technology and still an advisor at Kropman, introduced us to the Eindhoven Engine OpenCall. Recognizing its potential to foster meaningful partnerships, we eagerly seized this opportunity.
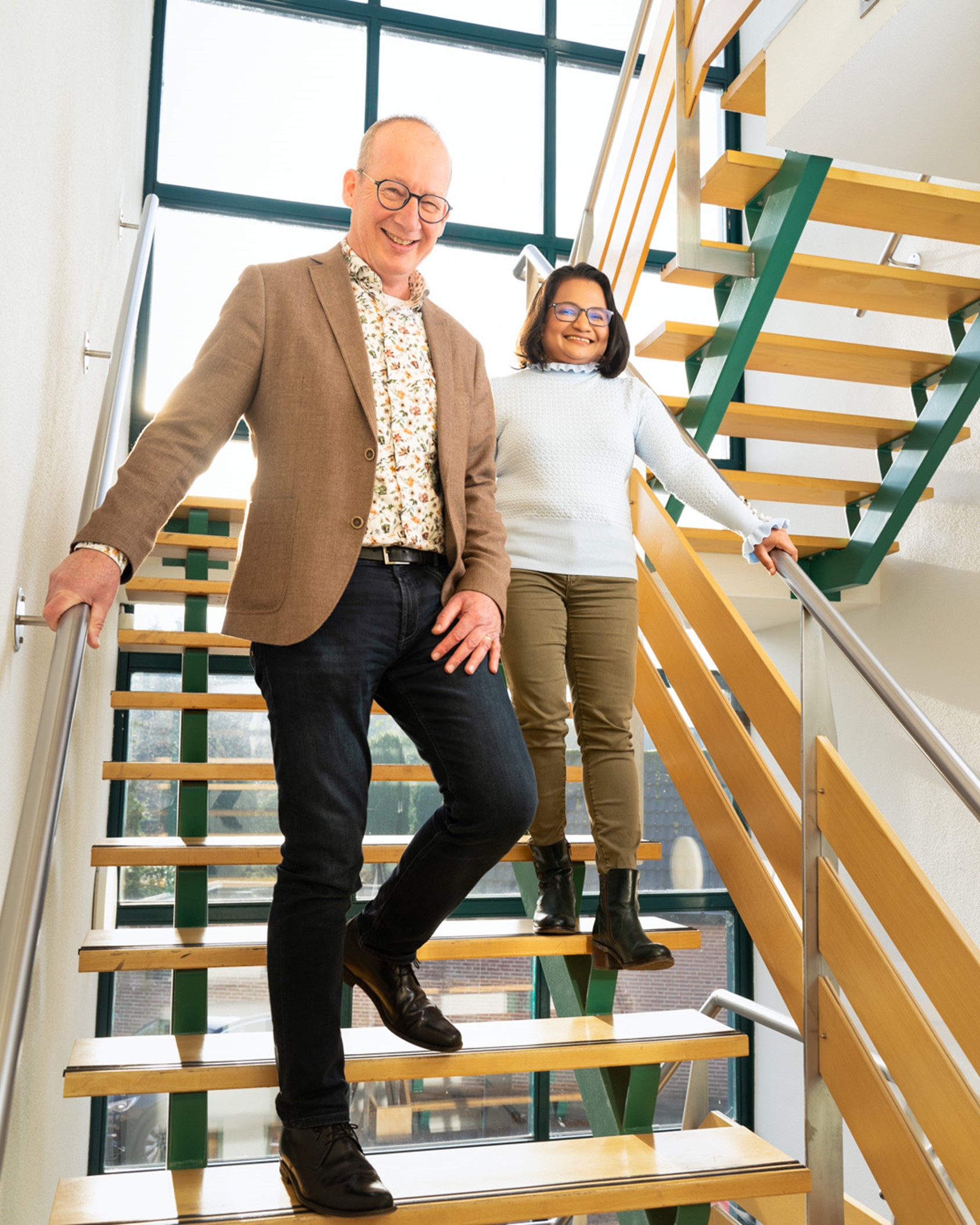
Eindhoven Engine’s OpenCall: A Catalyst for Collaboration
“Working with Eindhoven Engine offers a unique experience,” Shalika explains. “We share a location with a variety of companies and students, all engaged in their own projects. This diversity is beneficial. It’s not just about casual connections, like chatting at the coffee machine; we also attend sessions to share and discuss project progress.
For instance, while working on a data prediction project, I overheard a researcher at Eindhoven Engine’s Festival of Disruption event discussing data usage in cancer research. It didn’t click immediately, but later, the idea struck me at home: we could collaborate and enhance our respective research.
These interactions open up new perspectives and inspire innovative thinking. “This collaborative atmosphere was the reason Kropman signed up for three projects in a row. One project focused on detecting and diagnosing faults in building climate systems. The second project involved personalized thermal comfort systems, allowing employees to adjust their workspace climate via a smartphone app. The third was an assessment of CO2 sensors in the market, evaluating their accuracy for reliable CO2 readings in schools.
Although these projects are completed within Eindhoven Engine, we continue to build on the research and improve the products developed.” “And that’s why we brought Shalika on board,” says Joep.
Media: Smart shed powers buildings year-round
Two dynamic startups, CALOSOL and Cellcius , on the TU/e campus are collaborating on a groundbreaking project to drive forward the energy transition. They integrate revolutionary heat wall panels with intelligent energy storage encapsulated within a specially designed shed – introducing the innovative Smart Heat Shed
This pioneering solution guarantees a reliable energy source for winter heating needs in residential or office spaces. To enhance the system’s efficiency, an experimental setup has been established at Eindhoven Engine on the TU/e Campus. The project is one of 28 projects of Eindhoven Engine and was partly made possible by Regio Deal Brainport Eindhoven.
Source: Brainport Eindhoven
Media: ‘Slim schuurtje’ voorziet gebouwen het hele jaar door van energie (DUTCH)
Op de TU/e Campus in Eindhoven werken twee startups aan een nieuwe ontwikkeling die de energietransitie moet helpen versnellen. De startups combineren zogeheten warmtegevelpanelen met slimme energieopslag in de vorm van een berging; de zogeheten Smart Heat Shed.
Daardoor is bijvoorbeeld ook ‘s winters voldoende energie beschikbaar om een woning of kantoorpand te verwarmen. Om het systeem verder te verbeteren is een proefopstelling gebouwd op de TU/e Campus. Het project is één van 28 projecten van innovatieaccelerator Eindhoven Engine en is mede mogelijk gemaakt door Regio Deal Brainport Eindhoven.
Source: Brainport Eindhoven
Possible continuation for SmartMan project at Fontys
The SmartMan project at Eindhoven Engine is approaching its end date. SmartMan is an OpenCall project that started in 2020 and has a duration of three years. This summer, the project ends at Eindhoven Engine, but is working on a follow-up at Fontys Engineering.
Hans Krikhaar, who is leading this project and is a lecturer in Smart Manufacturing at Fontys Engineering, understood that SMEs have a great need for innovation, but lack the capacity and knowledge to do these innovations themselves. To be ahead of the competition, it is extremely important for SMEs to keep innovating their production processes. The industries need to adopt Smart Manufacturing. The fresh perspective and knowledge of Fontys students are the outcome here. In the ecosystem knowledge exchange at Eindhoven Engine makes cross-pollinations possible between Fontys students, SMEs, other companies and institutes. With the help of Eindhoven Engine, it results in a validated approach to strengthen the innovation power of SMEs in the Brainport region.
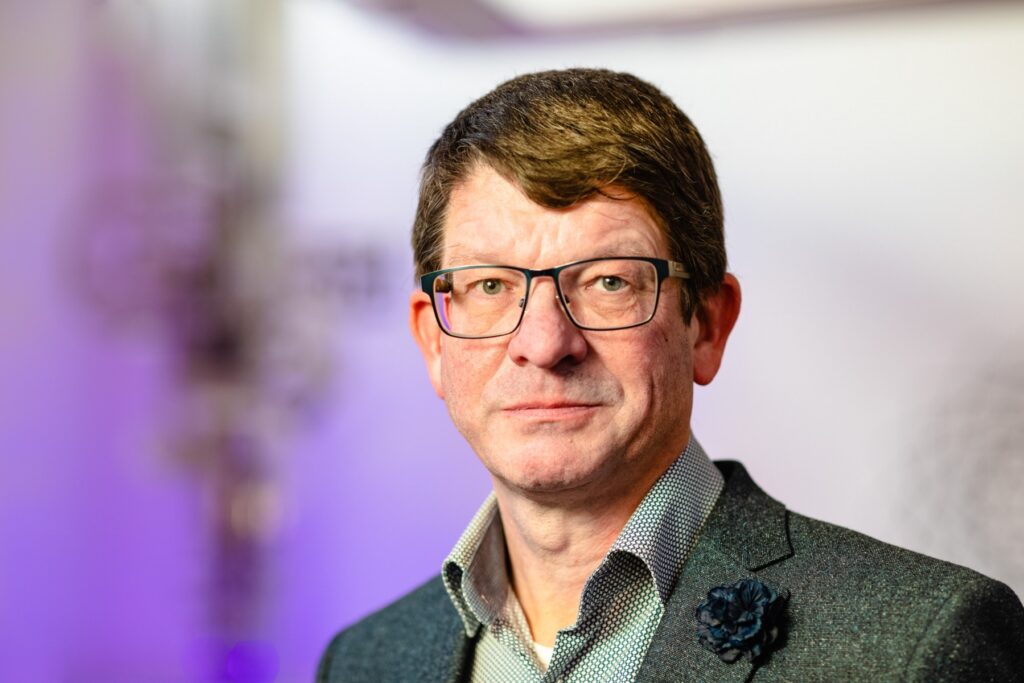
Projects in three focus areas
The goal of SmartMan project was to annually realize 40 subprojects carried out by 2nd, 3rd and 4th year Fontys students at SMEs. During the 3-year period at Eindhoven Engine, indeed a total of 120 projects at various companies were successfully completed. A very nice result considering this project withstood the corona epidemic. In the SmartMan project with Eindhoven Engine, Fontys, TNO and Brainport Industries as partners, students do their internship and graduation assignments in various facets of Smart Manufacturing.
These can be divided into three focus areas:
1. Topological design, which includes additive manufacturing and lightweight structures.
2. Intelligent systems (AI, low-code modeling and digital twinning).
3. Factory automation and robotics.
Knowledge exchange at Eindhoven Engine makes cross-pollinations possible between Fontys students, SMEs, other companies and institutes.
At VDL, successive students have worked on modular injection molds (topological design). In the area of intelligent systems, several students have used artificial intelligence for VBTI to further develop vision analysis, and at Siemens, students have collaborated on developing digital twins. Students have also contributed to factory automation solutions at various companies. At Eindhoven Engine project Carbyon DAC, a student even collaborated on the process plant for making CO2-absorbent material used to reduce CO2 in the atmosphere.
Stronger link
Fontys Engineering is working out plans to follow up on SmartMan. The goal is to link research even more closely to student projects in companies, especially in SMEs. Students will receive assignments within research domains at companies and teacher-researchers will provide the necessary knowledge transfer. This allows future students to complete their assignments more efficiently with the guidance and knowledge of the lecturer-researcher.
Possible continuation for SmartMan project at Fontys
The SmartMan project at Eindhoven Engine is approaching its end date. SmartMan is an OpenCall project that started in 2020 and has a duration of three years. This summer, the project ends at Eindhoven Engine, but is working on a follow-up at Fontys Engineering.
“I envision this configurator as something we will use here at Sorama to configure our products for different settings, for example smart stadiums, busy streets and probably more.” Devansh’s prior educational experiences include a BSc in Computer Science & Engineering from Punjab Engineering College, Chandigarh, India, and a MSc in Sound and Music Computing from Aalborg University Copenhagen, Denmark. He has also worked in various IT roles with firms like JPMorgan Chase, Shell and Bosch.
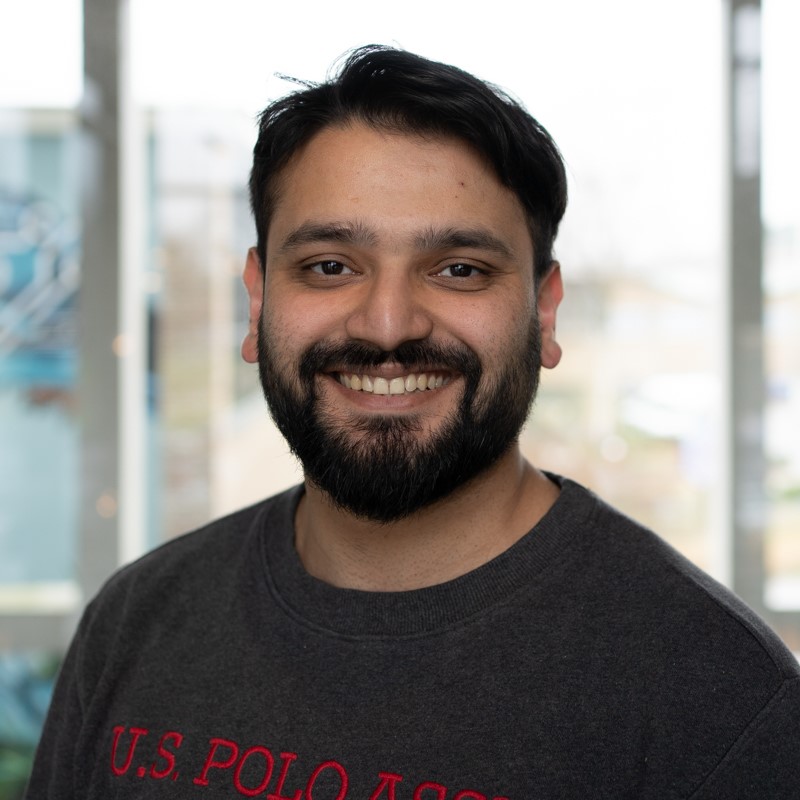
“I’m glad to be a part of this exciting setup here in Eindhoven,
and I look forward to meeting you soon!”
Devansh Kandpal I EngD trainee VIPNOM project
Possible continuation for SmartMan project at Fontys
The SmartMan project at Eindhoven Engine is approaching its end date. SmartMan is an OpenCall project that started in 2020 and has a duration of three years. This summer, the project ends at Eindhoven Engine, but is working on a follow-up at Fontys Engineering.
In 2019, I started my PhD, after which we initiated a clinical study called Neurotrend in collaboration with Philips and the epilepsy centre Kempenhaeghe. Neurotrend is one of the first Eindhoven Engine OpenCall projects.
Predicting the clinical outcome
This study is aimed at predicting the clinical outcome (i.e., the course/development of a disease) of people with depression based on MRI scans. More specifically, we obtain structural, functional (activity) and vascular MRI scans of the brain of subjects with and without depression at the beginning of the study and after a year. During the one-year period, we monitor their depression symptoms and cognitive ability. In this way, we can predict how the depression will develop over time based on the first scans but also evaluate brain changes over a year and correlate this to symptom changes. The clinical study was ethically approved in 2021 and its data acquisition is almost finished at the time of writing.
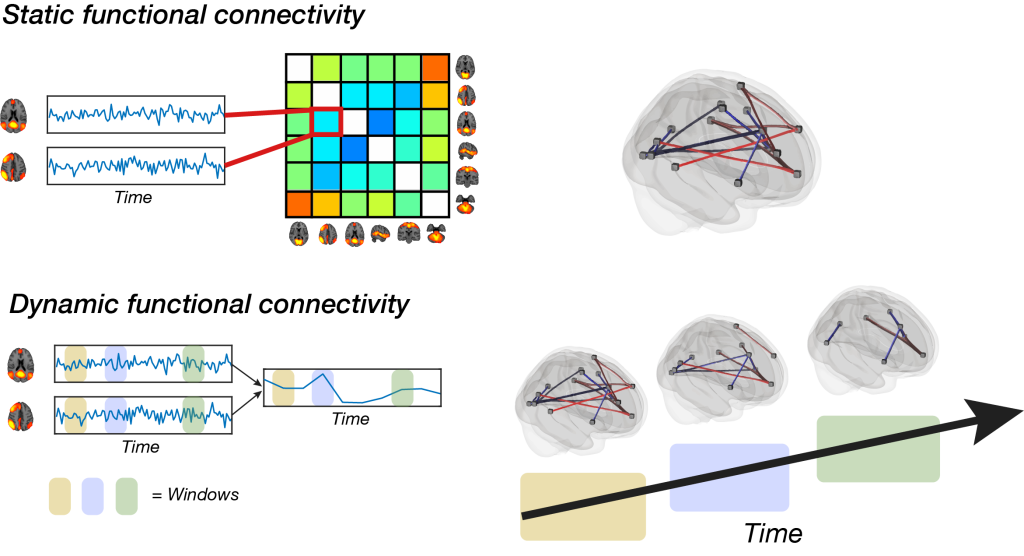
Preliminary results
From the preliminary results, we can conclude that brain activity patterns and interaction between brain networks is time-varying and that including this neurodynamic nature in a model improves the prediction of depression symptom severity changes over time compared to more standard/static approaches (brain activity/synchronicity over the whole functional MRI scan). Moreover, we demonstrate that a relatively novel MRI acquisition method, called multi-echo multiband imaging, increases the functional MRI signal quality and improves, amongst other things, the temporal resolution. This is beneficial as it allows us to more reliably model network interactions. Another interesting finding was the fact that brain volume and tissue properties of several limbic structures, which are known to be involved in emotion processing, also have predictive value for clinical outcome in depression. A smaller amygdala (associated with fear processing) volume correlated significantly with a higher number of lifetime depressive episodes.
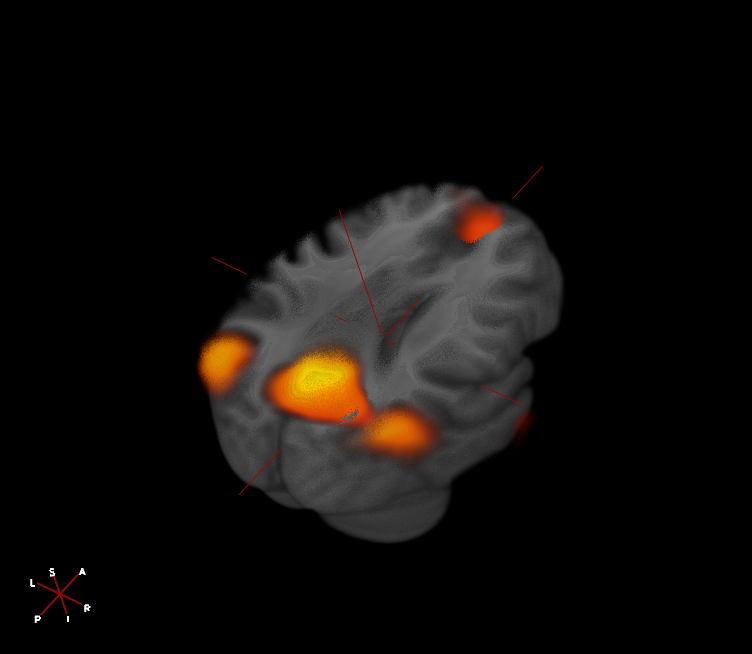
Improving the models and interpreting clinical meaning
In the last period of the PhD, I will focus on improving the models and interpreting the clinical meaning of these results, which will further help in understanding the aberrant brain mechanisms in subjects with depression. We hope to show other researchers the direction in which we think future MRI studies related to psychiatric disorders should head. Taking into account the complex, dynamically interactive brain while implementing the aforementioned MRI acquisitions could lead to more replicative results, especially if carried out in studies with a larger sample size. Even though we will not yet be able to apply these models in the clinic to support (still subjective) clinical decision-making, we are contributing significantly to existing depression-related MRI research. We have demonstrated the potential of state-of-the-art analyses and acquisitions in combination with a multi-modal MRI-based longitudinal study for depression diagnosis/prognosis purposes.