Project updates
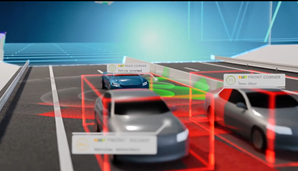
An update on the Smart Mobility project
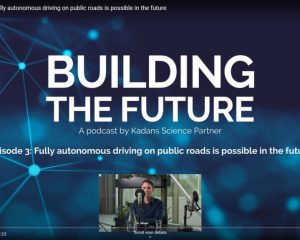
Building the Future: Fully autonomous driving on public roads is possible in the future
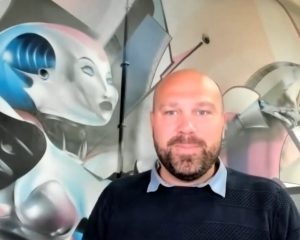
Smart Mobility @Eindhoven Engine during DTW
With the support from Eindhoven Engine, this project successfully hired EngD trainees and TAs, enhancing the project’s overall impact. The team also benefited from using the garage in Disruptor, which served as a collaborative workspace for developing the self-driving research vehicle. This co-creation space also served as a showroom, where demonstrations were given to stakeholders and external parties.