Stricter regulations
Air handling units (AHUs) are highly customized equipment. The regulations concerning AHUs are becoming increasingly strict to meet higher energy efficiency and ventilation goals, which adds to the complexity inherent within customized equipment. This upsurge in complexity increases the need for the continuous maintenance and monitoring of AHUs. However, such programs are difficult to implement due to the shortage of skilled personnel. Therefore, continuous monitoring and fault detection and diagnosis (FDD) processes need to be automated, which is referred to as AFDD. Despite the plethora of research on AFDD, there are limited real-life applications. Adding to this, the available solutions are either unreliable, unaffordable and/or unscalable.
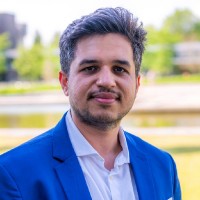
FDD tools
A survey of the literature on FDD tools (commercially deployed or under development) revealed that these tools rely on a combination of expert rules or first principles. These approaches are inherently reliant on sensed information and/or expert knowledge, which makes their sustainment untenable post-deployment. Moreover, such tools have a very limited ability to prevent significant energy wastage. It is estimated that up to 30% of energy could be saved by effectively using data collected with continuous monitoring systems.
Artificial Intelligence
To realize this potential, highly sensitive fault diagnosis models can be trained with Artificial Intelligence (AI) based approaches that are scalable and have less reliance on expert knowledge and sensors. Through this project, an AFDD tool that incorporates these approaches has been developed and tested at two buildings. Within the AI domain, gradient boosting techniques have been employed for fault detection and its diagnosis is handled using Bayesian networks. Through experimental validation, a diagnosis specificity exceeding 90% has been realized. Importantly, it has been observed that the prototyped AFDD tool could reduce the energy consumed in the deployed chiller by 33%.
*Continuous Monitoring and Fault Detection Diagnosis of large Heat Ventilation and Air Conditioning systems.
“It is estimated that up to 30% of energy could be saved by effectively using data collected with continuous monitoring systems.”